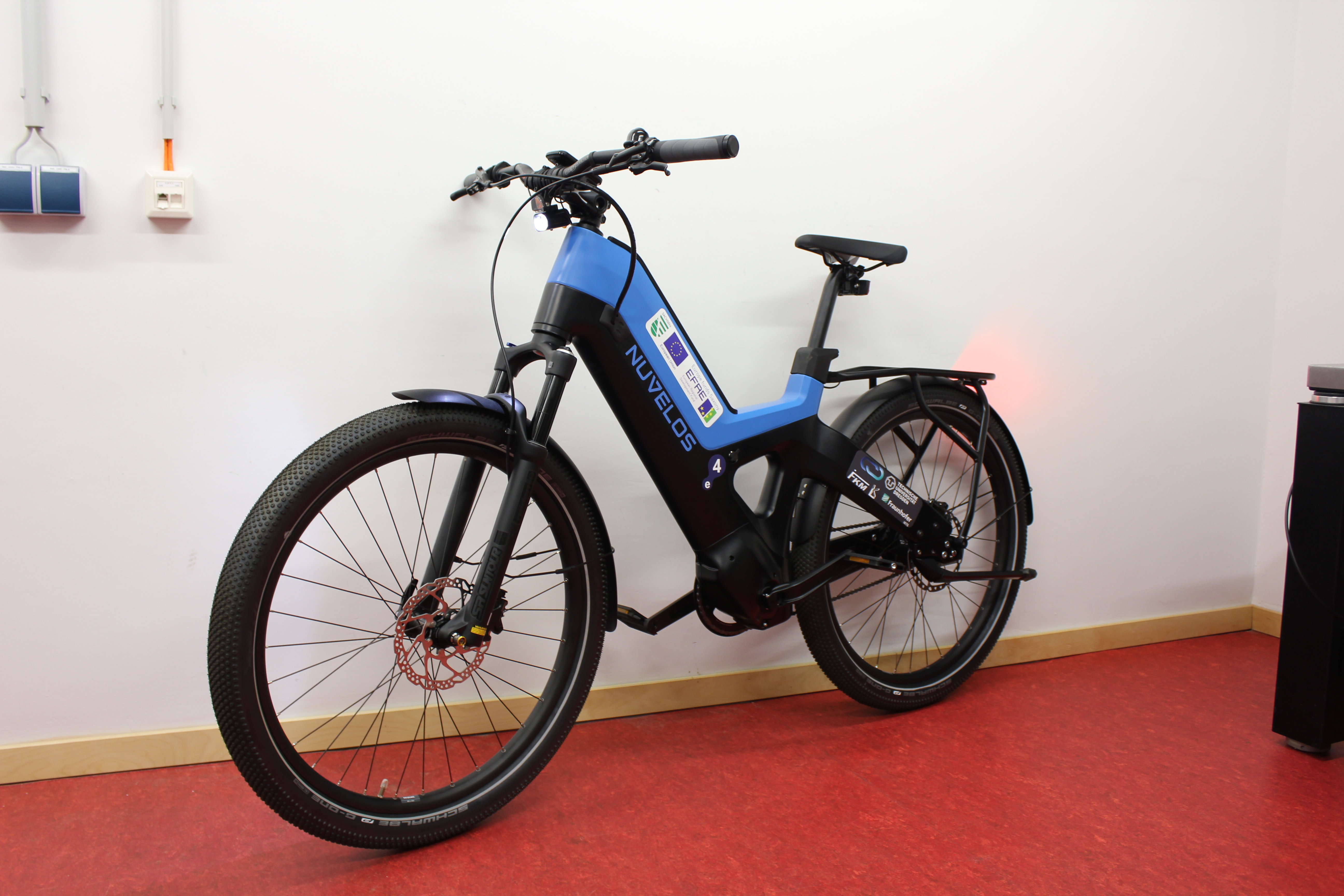
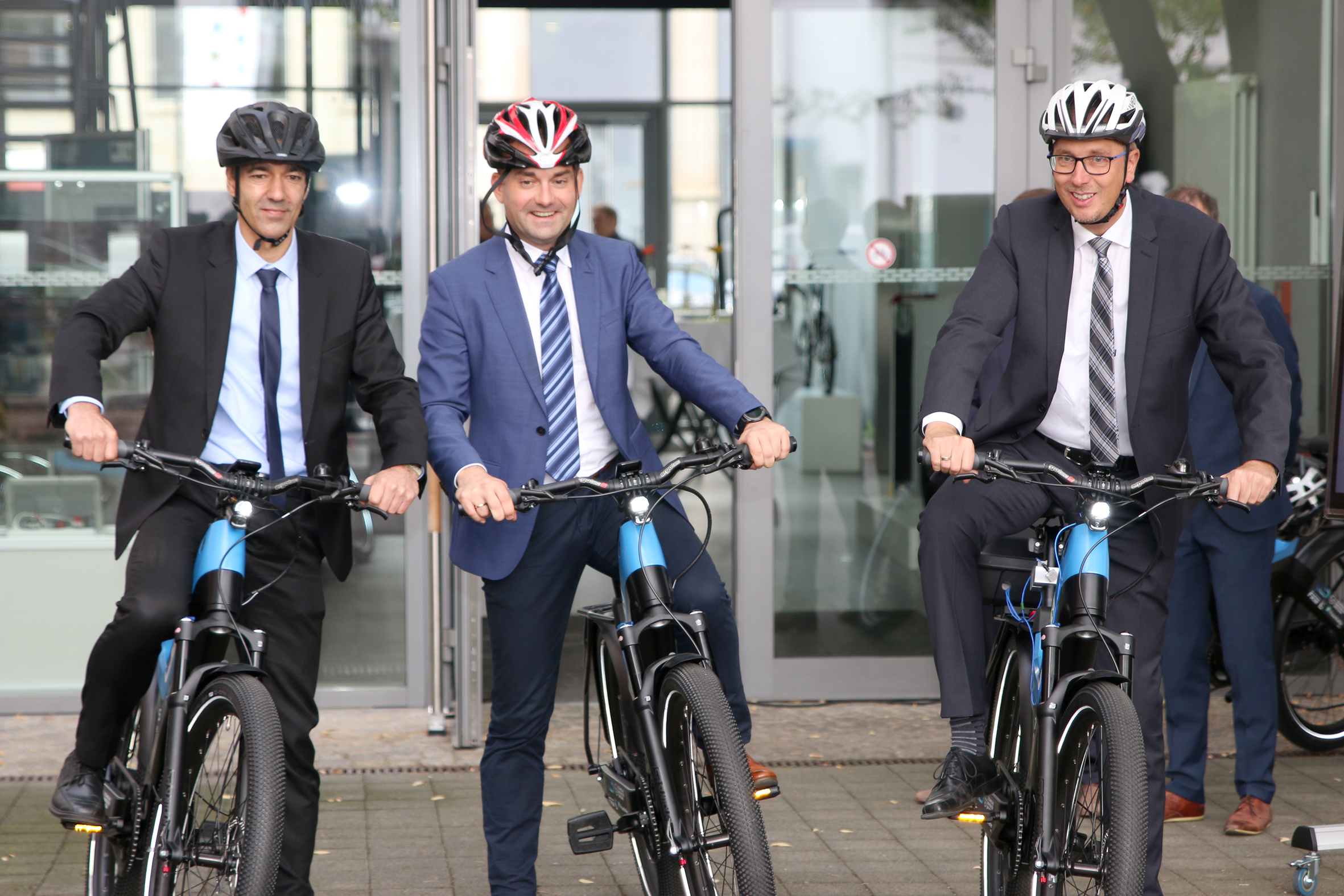
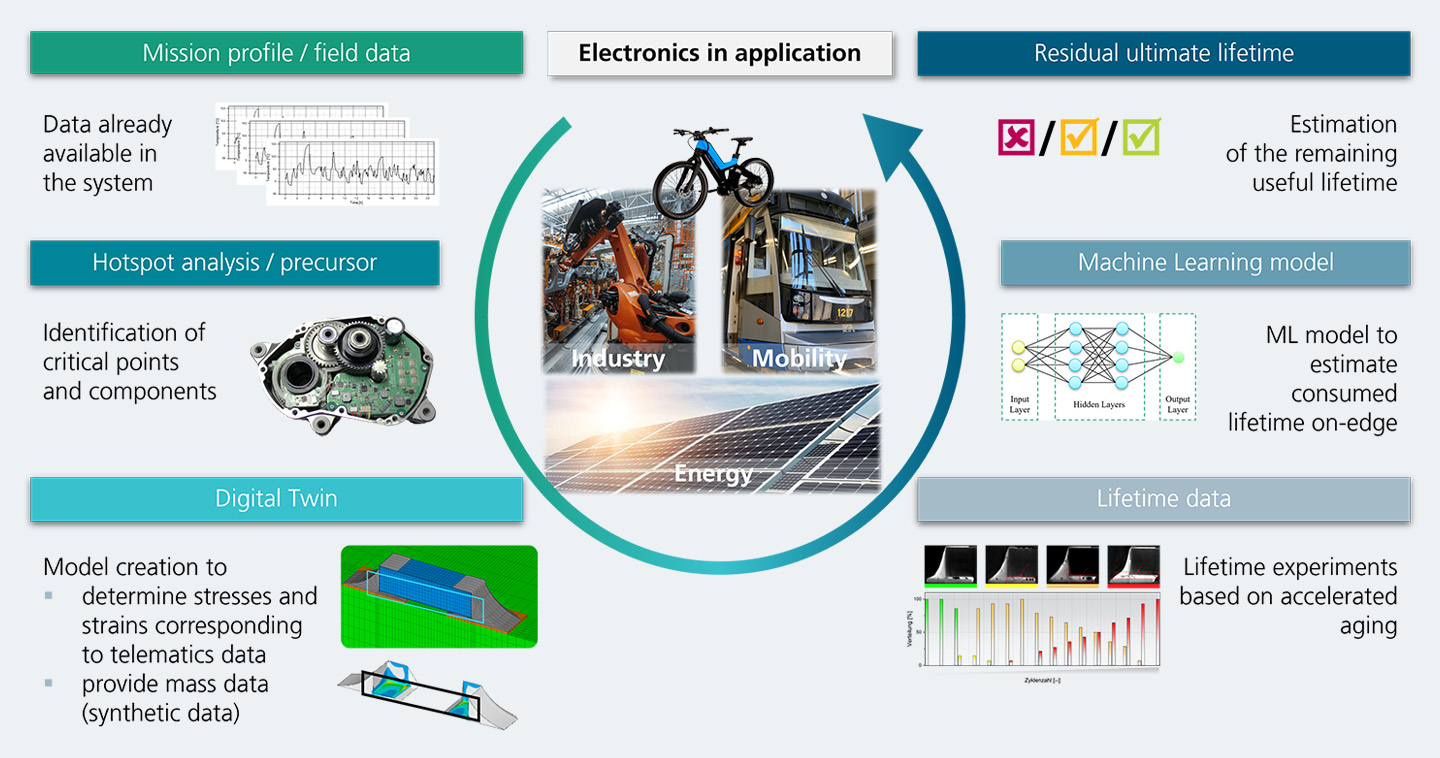
Two current trends that will also fundamentally change Saxony's society and industrial landscape are alternative mobility concepts and advancing digitalization.
Sustainable mobility requires new drive concepts that are as emission-free as possible. To increase the energy efficiency and range of electric drives, a further increase in power densities is required. This is achieved by new types of components that combine electronics and structure in compact dimensions. High thermomechanical loads occur – for example, due to the high packing density or also due to new operating modes, such as the recuperation of drive energy through electric braking.
In a joint research project with the Institute of Solid Mechanics (IFKM) and the Institute of Lightweight Engineering and Polymer Technology (ILK) at the Technische Universität Dresden, methods for linking collected measurement data with service life forecasts of highly integrated mechatronic systems will be developed over the next three years.
A fleet of e-bikes with thermoplastic frame construction, which were co-developed by the ILK in previous research projects, will serve as measurement vehicles. These will be used to collect large volumes of measurement data on thermomechanical and electrical stress, store this data in a data cloud and evaluate it using artificial intelligence methods for condition prediction and service life forecasting. In parallel, model-based simulations of the bicycle frame and essential electronic elements are being created. The results are used to expand the experimental database, e.g., regarding untested driving conditions and extreme situations.
Funding: Resources of the European Union ERDF fund
Project timeframe: August 2019 – June 2022
Project partners: Technische Universität Dresden, Institute of Solid Mechanics (IFKM) and Institute of Lightweight Engineering and Polymer Technology (ILK)